What Are the Future Trends in Big Data and Analytics?
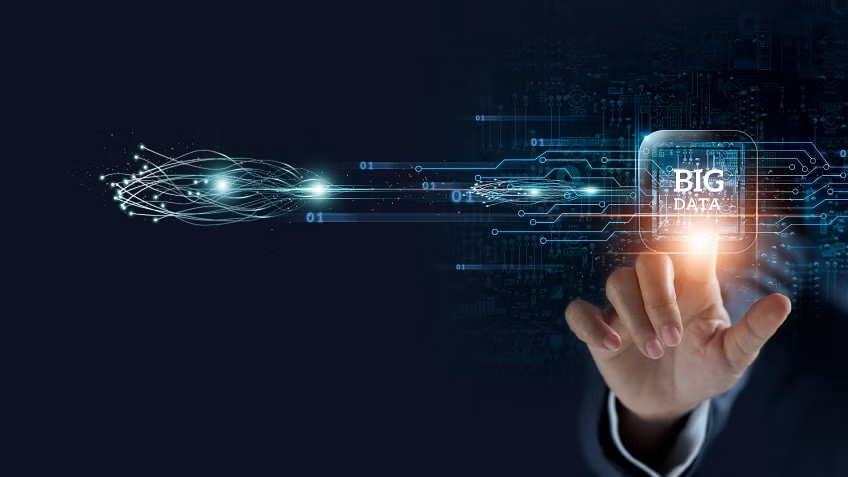
In today’s rapidly evolving digital landscape, big data and analytics are playing an increasingly central role in shaping industries and driving innovation. Businesses are collecting vast amounts of data from a variety of sources, ranging from social media to IoT devices, and leveraging advanced analytics to turn this data into actionable insights. As technology continues to advance, the future of big data and analytics promises to bring even more powerful tools, innovations, and applications that will redefine how businesses operate and make decisions.
This article explores the future trends in big data and analytics, how these trends are likely to influence various industries, and how organizations can prepare for these changes to stay competitive.
1. The Growth of Data Volume and Variety
The volume, variety, and velocity of data are increasing at an exponential rate. As businesses and consumers generate more data than ever before, the sheer amount of information being collected is growing rapidly. According to industry reports, global data creation is projected to grow to over 180 zettabytes by 2025. This flood of data is coming from a wide array of sources, including IoT devices, social media, transactional systems, and digital platforms.
In the future, companies will need increasingly sophisticated data management solutions to handle and analyze such vast amounts of diverse data. Technologies like data lakes, cloud storage, and advanced data processing frameworks will become more important as organizations need to store, process, and analyze structured and unstructured data in real-time. Moreover, the ability to extract meaningful insights from such diverse data sources will be crucial for businesses to maintain a competitive edge.
2. Artificial Intelligence and Machine Learning Integration
Artificial Intelligence (AI) and Machine Learning (ML) are poised to be the backbone of big data and analytics in the future. As AI and ML technologies continue to evolve, they will play an even more prominent role in analyzing and interpreting vast datasets with speed and accuracy that surpasses human capabilities.
Machine learning algorithms will be able to identify patterns and trends within large datasets that would be impossible for human analysts to discern. As a result, predictive analytics will become even more accurate, enabling businesses to make data-driven decisions that improve outcomes. AI can also automate complex tasks in data processing and analysis, such as data cleaning and feature engineering, freeing up human analysts to focus on higher-level decision-making.
Furthermore, AI-powered algorithms will allow for real-time decision-making. Whether it’s optimizing supply chains, personalizing marketing strategies, or detecting fraud in financial transactions, AI and ML technologies will become integral to business operations, improving efficiency and driving innovation.
3. Real-Time Data Analytics
As businesses increasingly rely on fast and agile decision-making, the ability to process and analyze data in real-time will become a critical competitive advantage. Real-time data analytics will enable businesses to respond immediately to emerging trends, consumer behaviors, and market shifts.
For example, industries like e-commerce and retail can use real-time analytics to personalize customer experiences, detect changes in buying behavior, and optimize pricing strategies. In manufacturing, real-time analytics can be used for predictive maintenance, ensuring that equipment failures are prevented before they cause downtime.
The demand for real-time analytics will also increase the need for edge computing. Edge computing allows data to be processed closer to the source, such as on IoT devices, before being transmitted to centralized data centers. This reduces latency and enables faster insights, ensuring that businesses can act on data immediately, rather than waiting for it to be processed in a cloud environment.
4. Cloud Computing and Hybrid Cloud Solutions
Cloud computing has already transformed the way businesses manage and store data, but as big data continues to grow, cloud infrastructure will play an even greater role. Cloud providers offer scalable, cost-effective solutions that allow companies to store and process large volumes of data without investing heavily in on-premise infrastructure.
In the future, hybrid cloud solutions—where businesses use a combination of private and public cloud services—will become more prevalent. These solutions offer flexibility, allowing businesses to store sensitive data on private clouds while leveraging the scalability of public clouds for less sensitive information. The ability to dynamically scale resources based on data requirements will be crucial for businesses managing large datasets in a secure and cost-effective manner.
Moreover, as cloud platforms continue to innovate, the integration of cloud-native analytics tools will allow businesses to seamlessly analyze big data using advanced AI and ML models without the need for complex infrastructure setups.
5. Data Privacy and Security
As data volumes grow, concerns surrounding data privacy and security are becoming more critical. With increasing regulatory scrutiny and consumer concerns about how their data is being used, businesses must ensure that they are compliant with data protection laws such as the GDPR (General Data Protection Regulation) in the EU and CCPA (California Consumer Privacy Act) in the US.
In the future, the demand for advanced data security technologies will rise, especially as organizations rely on big data for decision-making. Techniques like encryption, anonymization, and tokenization will become more commonplace, ensuring that data can be analyzed while keeping sensitive information protected.
Additionally, the use of blockchain technology may also gain traction in ensuring data security and transparency. Blockchain can create immutable records of data transactions, providing an extra layer of protection against tampering and ensuring the integrity of big data in industries such as finance and healthcare.
6. Automated Data Analytics
The complexity of analyzing big data will drive the need for more automated tools that simplify the process. Future big data analytics platforms will leverage AI and automation to allow businesses to perform analytics with little to no human intervention. These platforms will use automated data discovery, anomaly detection, and pattern recognition to quickly generate insights without requiring analysts to manually set up complex queries.
This trend will democratize data analytics by making it more accessible to organizations of all sizes and skill levels. Businesses that lack extensive data science teams will be able to use automated analytics platforms to uncover valuable insights and make informed decisions. Furthermore, the use of automated tools will increase efficiency, reduce human error, and allow organizations to scale their analytics efforts more effectively.
7. Democratization of Data
As data becomes a key driver of business decisions, it is essential that organizations make data more accessible to everyone within the organization, not just data scientists and analysts. The democratization of data will empower employees at all levels to make data-driven decisions and contribute to the organization’s overall success.
In the future, we can expect more user-friendly tools and dashboards that allow non-technical employees to explore data and generate insights. These self-service analytics tools will allow individuals to easily query and analyze data without needing to know complex SQL queries or statistical techniques. This trend will foster a data-driven culture within organizations, ensuring that all employees can leverage data to improve their work.
8. Artificial Intelligence for Data Governance
With the growing volume of data, managing its quality, security, and compliance will become increasingly complex. Artificial Intelligence (AI) will play a key role in data governance by automating tasks such as data classification, auditing, and compliance checks. AI systems will help organizations ensure that they are adhering to regulatory requirements and industry standards, reducing the risk of data breaches and non-compliance.
Additionally, AI will help identify data inconsistencies and anomalies, ensuring that organizations can trust the accuracy and integrity of their datasets. This will be particularly important as organizations rely on data for critical decision-making and strategic planning.
FAQs
1. What is the future of big data and analytics?
The future of big data and analytics involves integrating AI, machine learning, real-time data analysis, and advanced cloud solutions to help businesses make faster, data-driven decisions. Key trends include automation, data privacy, and improved data accessibility.
2. How will AI and machine learning impact big data?
AI and machine learning will enhance big data by automating data processing, identifying patterns, and generating actionable insights. These technologies will improve predictive analytics and enable businesses to make real-time decisions.
3. What role will cloud computing play in big data analytics?
Cloud computing will continue to provide scalable, cost-effective infrastructure for managing and analyzing big data. Hybrid cloud solutions will become more popular, offering flexibility and security for businesses handling large datasets.
4. How can organizations protect data privacy while leveraging big data?
Organizations will need to adopt advanced security measures, including encryption, anonymization, and data tokenization, to ensure data privacy while maintaining compliance with privacy regulations like GDPR and CCPA.
5. What is automated data analytics, and how does it work?
Automated data analytics uses AI and machine learning to simplify the process of analyzing data. It allows businesses to perform complex analyses and uncover insights without the need for human intervention, improving efficiency and scalability.
6. How can businesses make data more accessible?
The democratization of data involves creating user-friendly analytics platforms that allow employees at all levels to access, explore, and analyze data. This empowers employees to make data-driven decisions without needing technical expertise.
7. Why is real-time data analytics important?
Real-time analytics enable businesses to act immediately on data, responding to emerging trends, customer behaviors, and market shifts. This leads to more agile decision-making and improved customer experiences.
Conclusion
The future of big data and analytics promises a world of possibilities. With the rapid growth of data and technological advancements, businesses will increasingly rely on AI, machine learning, and real-time analytics to make faster, data-driven decisions. Cloud computing and hybrid cloud solutions will play a central role in managing vast datasets, while data privacy and security will remain top priorities.
Organizations that embrace these future trends will be better positioned to leverage the power of big data to drive innovation, improve operational efficiency, and create new revenue streams. As big data continues to evolve, businesses must stay ahead of the curve to remain competitive in an increasingly data-driven world.
Key Takeaways
- Big data and analytics will continue to grow in importance as data volumes increase and business needs evolve.
- AI and machine learning will be key drivers in enhancing predictive analytics, automating processes, and generating insights.
- Real-time data analytics and cloud computing will empower businesses to act quickly and efficiently.
- Data privacy and security will remain critical concerns, driving the demand for advanced governance tools.
- The democratization of data and automated analytics will allow organizations to leverage data insights at all levels, promoting a data-driven culture.
Leave a Comment